Challenges and Benefits of Predictive Analytics in Finance
The amount of information in the world today is growing rapidly in almost every business domain. The operation of financial institutions as well as the entire fintech industry is no exception. In order to find, receive and process important information in time in all this data flow, companies should use up-to-date capabilities and tools of data analysis systems. Predictive analytics is one of these tools.
By implementing this analytics tool efficiently allows companies to predict future results, understand market trends, and identify issues and bottlenecks that may remain unnoticed via traditional methods.
According to this study by Statista, the market for applications with implemented predictive analytics will reach $41.52 billion by 2028. Let’s take a closer look at this topic in our article and answer the question of what challenges may face companies from the financial field. We’ll also consider types and benefits of predictive analytics in finance.
Unveiling the Key Challenges in Finance
First, let’s identify the main challenges in the finance sector that predictive analytics can help overcome.
- Regulatory Compliance
There are a number of regulations that financial institutions must comply with to ensure consumer protection and prevent financial crime, including the Dodd-Frank Act in the USA and the General Data Protection Regulation (GDPR) in the EU. Proper following of these and other regulations requires deep legal knowledge and rigorous compliance reporting. - Volatility and Risk Management
Market volatility depends on a number of factors, such as geopolitical events, economic indicators, and natural disasters. This unpredictable nature of global markets can cause financial risks and losses, as well as make it hard to realize promising opportunities. - Loan Default Risks
For companies working with finance, accurate credit risk assessment and loan default management are critical indicators. Typically, such risks are calculated using credit scoring models that allow assessing the risk of loan default. Thanks to predictive analytics tools, this process can be automated and significantly accelerated, as well as increased in accuracy. - Financial Fraud and User Security
In a world where most financial services have become digital, there are many cyber threats such as phishing attacks, ransomware, identity theft, etc. Situations where financial institutions fail to protect themselves and their audiences from these types of threats can lead to significant financial losses, lower customer trust, and large fines from the government.
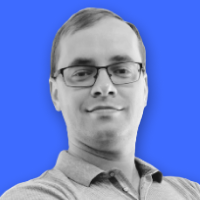
The Major Types of Predictive Analytics in Finance
In this chapter, we will look at the main types of predictive analytics that are widely implemented to deliver effective mobile and web development solutions for the market today.
Time Series
This type of predictive analytics focuses on analyzing and forecasting data that is collected over a certain period of time. A very illustrative example of how such a model works is a set of call center operations, which are still widely used in financial institutions.
Depending on office hours, days of the week, holidays, and other factors, the number of calls processed by the call center is uneven throughout the day. A time series model can significantly improve efficiency in such cases by using an Autoregressive Integrated Moving Average (ARIMA). With its help, it is possible to predict the approximate number of calls that financial organizations will receive per hour/day/week.
Having such information helps financial and other similar companies effectively plan their resources, distribute working hours, and ensure better call management.
Decision Tree
Decision tree algorithms are another powerful tool for operating predictive analytics in finance properly. They work by breaking data into branches, similar to the tree-like structure, to illustrate all possible results of each certain decision. This method aids in identifying the customer journey, allowing businesses to understand their clients better and come up with more personalized service.
Due to the visual representation of this model it’s quite simple to interpret the decision tree model’s results and understand how it gets its predictions. Companies often use the decision tree model in combination with financial solutions for credit risk assessment, fraud detection, client segmentation, and a few more similar purposes.
Neural Networks
Developed based on the structure of the human brain, neural networks are able to capture complex patterns and relationships in data. Because of the ability of neural networks to learn nonlinear relationships in data, financial services firms often use them to perform high-frequency trading, credit scoring, and other market metrics analysis.
Classification Model
Сompanies from the financial field often implement this approach to optimize business processes, reduce existing and potential risks, and improve customer service.
Thus, classification models can be used, in particular, to retain customers by predicting their churn or potential ways to attract them. Based on the static data, companies can predict which customers are most likely to stop using their products or services, as well as the ways to engage a potential audience. Based on this data, companies usually take specific measures to retain valuable customers and involve new ones.
Predictive Analytics in Finance: The Main Benefits
So, we have covered the most popular predictive analytics models that are widely used in the financial industry today. Hopefully, you have already found the one that suits your business needs best. If not, let’s look at the main benefits that predictive analytics may bring to your business operations.
- Risk Mitigation
With the help of predictive analytics tools, financial companies can study large amounts of data to prevent potential fraud, identify vulnerabilities into their systems, and significantly reduce financial losses. The combination of such activities affects the entire risk management process. - Revenue Growth
Using the appropriate data analytics tools helps companies to positively impact the revenue level. With these tools, financial companies usually optimize pricing strategies and identify cross-selling and upselling opportunities. This, in turn, maximizes sales options and increases revenue generation methods, as well as leads to increased customer engagement. - Budgeting and resource allocation
With predictive analytics tools, companies can identify patterns and trends in data from multiple sources and predict the estimated return on investment. By analyzing large amounts of financial data, predictive analytics technology can suggest the most efficient ways to allocate resources and avoid overspending. - Personalized Customer Service
By studying various types of customer and financial market data, companies in this field gain an in-depth understanding of both their customer profiles and the overall market dynamics. Based on this analysis, companies are able to provide personalized financial tools and services, as well as financial management recommendations and investment strategies. This approach builds trust with the audience and forms long-term relationships.
